Cognitive warfare is reshaping modern conflicts by targeting perceptions, emotions, and decision-making rather than relying on traditional military force. This strategic manipulation destabilizes societies, exploits cultural narratives, and influences global power dynamics. To counter this, advanced AI, including Large Language Models (LLMs), offers a solution by detecting subtle narrative shifts, analyzing sentiment, and enhancing strategic decision-making to navigate complex geopolitical landscapes with greater precision and adaptability.

What is Cognitive Warfare?
Cognitive warfare is an emerging form of conflict that targets the psychological, cultural, and informational dimensions of societies rather than relying solely on traditional military or kinetic force. It involves manipulating perceptions, beliefs, emotions, and decision-making processes of populations to influence political, social, or military outcomes. In contemporary geopolitics, cognitive warfare is employed extensively by nation-states to shape narratives, amplify societal divisions, and exploit cultural or historical grievances.
The implications of cognitive warfare are profound, as they can destabilize democracies, fuel extremism, or undermine social cohesion without direct military engagement. Examples include Russia's use of sophisticated influence methods, exemplified by activities uncovered in Operation Secondary Infektion, while China has strategically influenced narratives surrounding sensitive geopolitical issues, notably through leveraging historical memories such as the Century of Humiliation, shaping domestic and international perceptions on sensitive geopolitical issues like Xinjiang and Hong Kong.
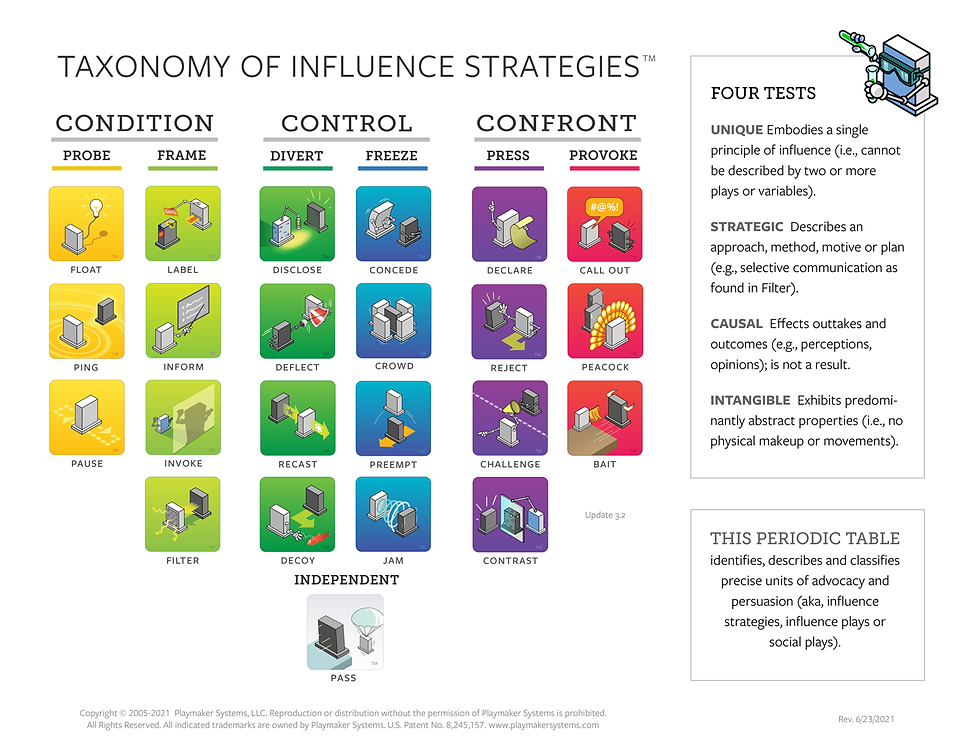
In a geopolitical landscape increasingly defined by subtle manipulation and strategic perception management, cognitive warfare has become a critical domain of modern conflict. Unlike traditional warfare, cognitive warfare targets the psychological and cultural dimensions of societies, exploiting deep-rooted beliefs, cultural identities, and historical experiences. Conventional approaches relying heavily on linear analysis and rigid frameworks often prove inadequate when faced with the complexities inherent in understanding and engaging with the nuanced reasoning styles characteristic of cultures such as China and Russia.
The U.S. and its Western allies frequently employ a linear reasoning style, interpreting truth in binary terms. Conversely, Chinese philosophy, rooted in Classical Chinese dialectical traditions—contrasting with modern Western binary logic—tends to interpret truth as contextual, situational, and inherently flexible. This fundamental disconnect between cognitive frameworks creates significant vulnerabilities in intelligence analysis, diplomatic strategies, and military operations.
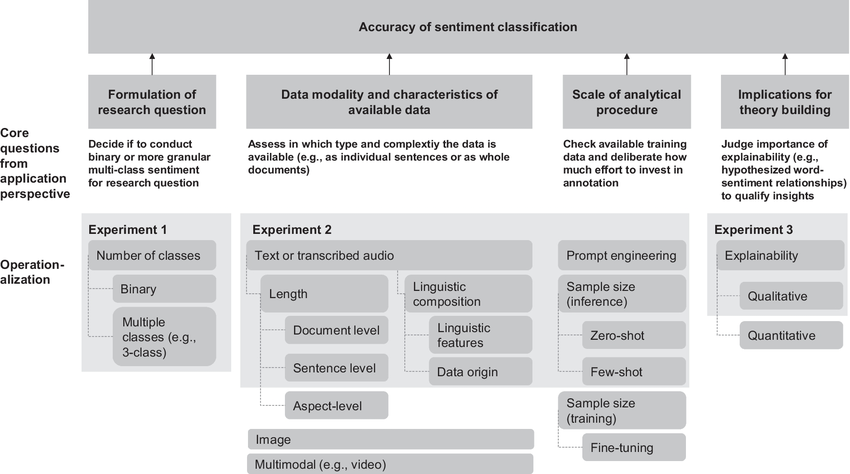
Large Language Models (LLMs) and machine learning offer significant potential to bridge these cognitive divides. Specifically, LLMs can process sentiment analysis and detect subtle narrative shifts often missed by human analysts, particularly in contexts where historical trauma, such as colonization or prolonged geopolitical conflicts, influences contemporary perspectives. Integrating advanced ML tools into strategic planning can provide deeper insights into how populations perceive truth, legitimacy, and authority.
A practical application of LLMs involves capturing underlying sentiments by identifying metaphorical language and quantifying nuanced yet crucial shifts in domestic and international narratives. These capabilities enable decision-makers to predict societal reactions, preempt counter-narratives, and develop culturally resonant communication strategies.
However, the effective deployment of these tools involves significant challenges. Ensuring data accuracy and relevance is essential for generating valid insights. Developing robust, adaptable models that can dynamically interpret complex narratives also poses substantial difficulties. Most importantly, bridging the gap between technical expertise and deep cultural and historical knowledge remains crucial. Addressing biases in training data and maintaining compliance with ethical norms and international standards further underscores the need for interdisciplinary collaboration.
Looking Ahead
Moving forward, an effective AI strategy for cognitive warfare must be intentional, agile, and well-calibrated. Expanding model training with culturally rich datasets will improve narrative interpretation accuracy, while a proactive approach to identifying and mitigating biases within algorithm architectures will help preserve credibility. Cross-disciplinary collaboration—bridging technical, social, and policy expertise—will be essential for developing holistic solutions to complex geopolitical challenges. Additionally, establishing clear ethical and legal frameworks is crucial to ensuring responsible deployment in sensitive domains.
One of the greatest challenges in developing and deploying machine learning applications is the tendency to delay action in pursuit of an ideal scenario—perfect datasets, optimized pipelines, fully trained models, and comprehensive regulatory guidelines. However, this overly cautious approach often leads to missed opportunities to gain a strategic edge. Staying lean and agile in the early stages of technological development is crucial, as we are at a pivotal moment where iterative deployment can drive rapid improvements and strengthen competitive positioning.
China’s recent advancements in artificial intelligence highlight the power of combining state-backed initiatives with startup agility. The launch of DeepSeek in January 2025 disrupted the market with its advanced capabilities in enhanced reasoning and problem-solving, followed shortly by Manus, reportedly the world’s first fully autonomous AI agent, developed by Butterfly Effect. The company’s name itself reflects Chinese strategic thinking—the idea that small technological advances can trigger profound geopolitical shifts. These rapid developments demonstrate China’s ability to reshape industries and challenge the long-standing perception of the country as merely a manufacturing hub lacking technological and engineering innovation, underscoring the urgency for the U.S. to assert leadership and drive innovation in the AI industry.
These examples highlight the necessity of starting with proof-of-concept models, swiftly progressing to pilot implementations, and enabling early user interaction. An iterative, proactive approach fosters continuous improvement, accelerates the learning curve, and secures a crucial advantage in the fast-evolving domain of cognitive warfare. Waiting for perfect conditions is not a viable strategy—timely execution and adaptability will define success in this rapidly shifting landscape.
Reference:
[1] Kelly, A., & Sipe, R.,”Why responding is losing: The plays we run (and the plays we don’t) to defeat disinformation,” Small Wars Journal, January 18, 2022. Link
[2] Hartmann J, Heitmann M, Siebert C et al. (2023) More than a Feeling: Accuracy and Application of Sentiment Analysis. Link
[3] Ng, Lynnette H.X. & Carley, Kathleen M. (2022). Is my stance the same as your stance? A cross validation study of stance detection datasets. Information Processing & Management. Link
[4] Krugman, Jan O. & Hartman, Jochen (2024). Sentiment Analysis in the Age of Generative AI. Customer Needs and Solutions. Link
[5] Juros, Jana & Majer, Laura & Snajder, Jan (2024). LLMs for Targeted Sentiment in News Headlines: Exploring Different Levels of Prompt Prescriptiveness. Link
Cognitive Warfare in the AI Age: A Strategic Imperative
Disclaimer: The articles provided above serve as informational resources, but readers are encouraged to conduct further research and seek professional advice before making decisions based on the content. The views expressed in these articles are those of the authors and do not necessarily reflect the opinions of any organizations mentioned.
Comments